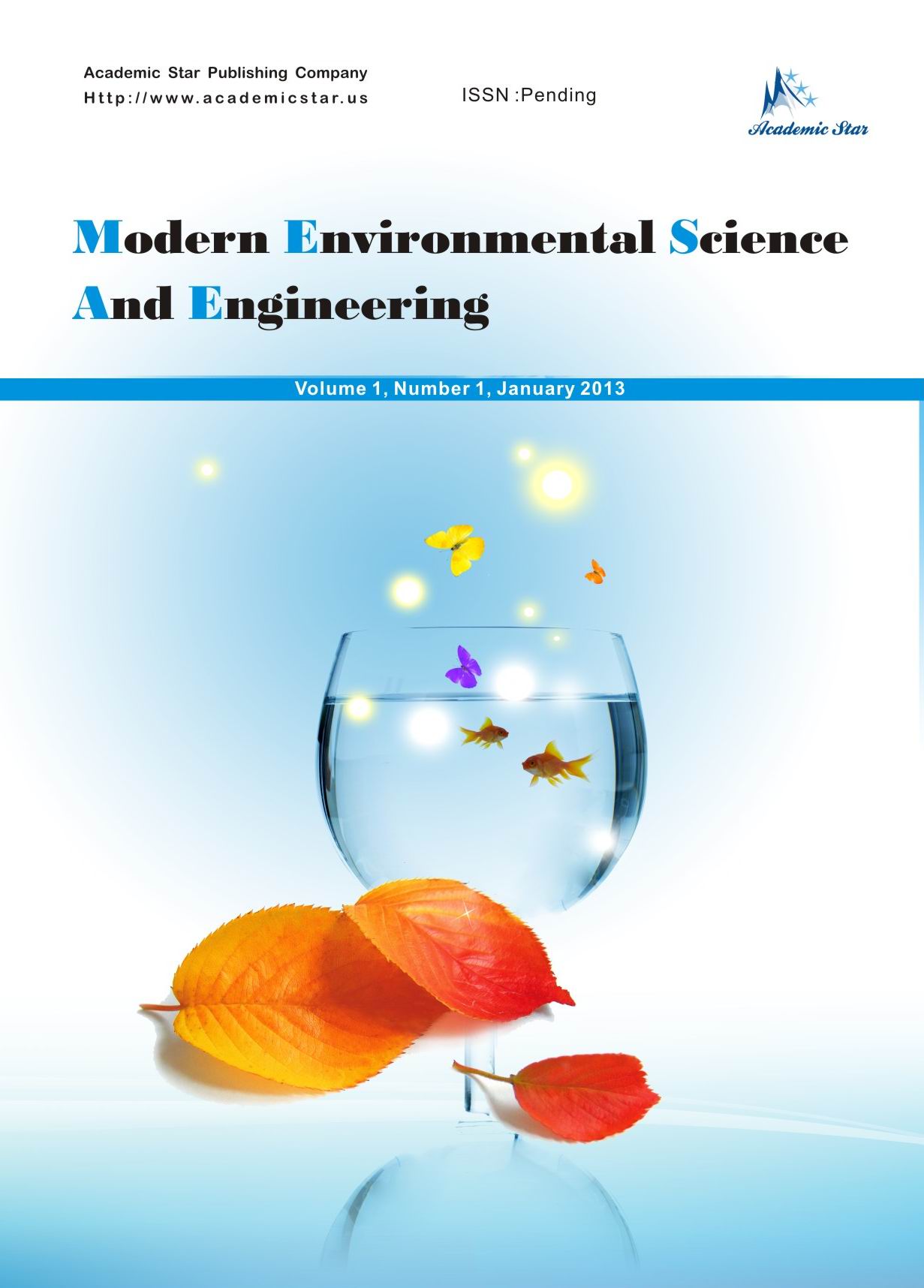
- ISSN: 2333-2581
- Modern Environmental Science and Engineering
Change Detection in Multitemporal Remote Sensing Images Using Support Vector Machines and Pixel Relevance
2. La Salle University – UNILASALLE, Brazil
Abstract: This paper investigates an approach to the problem of change detection in multitemporal remote sensing images using Support Vector Machines (SVM) based on RBF kernel (Radial Basis Function) combined with a new relevance metric called Delta b (Δb). The methodology is based on the difference of the fraction images produced for each date. In images of natural scenes the difference in soil and vegetation fractions tends to have a symmetrical distribution around the mean of its pixels. This fact can be used to model two normal multivariate distributions: change and non-change. The Expectation-Maximization algorithm is implemented for estimating the parameters (mean vector, covariance matrix, and prior probability) associated with these two distributions. Random samples are extracted from these two distributions and used to train a SVM classifier based on RBF kernel. The proposed methodology is tested using multi-temporal data sets of multispectral images Landsat-TM covering the same scene, located in Roraima state, in two different dates. Test samples are obtained by the use of Change Vector Analysis (CVA) and used to validate the estimation method of relevance. It is expected that this methodology could be applied to detection of change for multispectral and hyperspectral multitemporal images used in remote sensing.