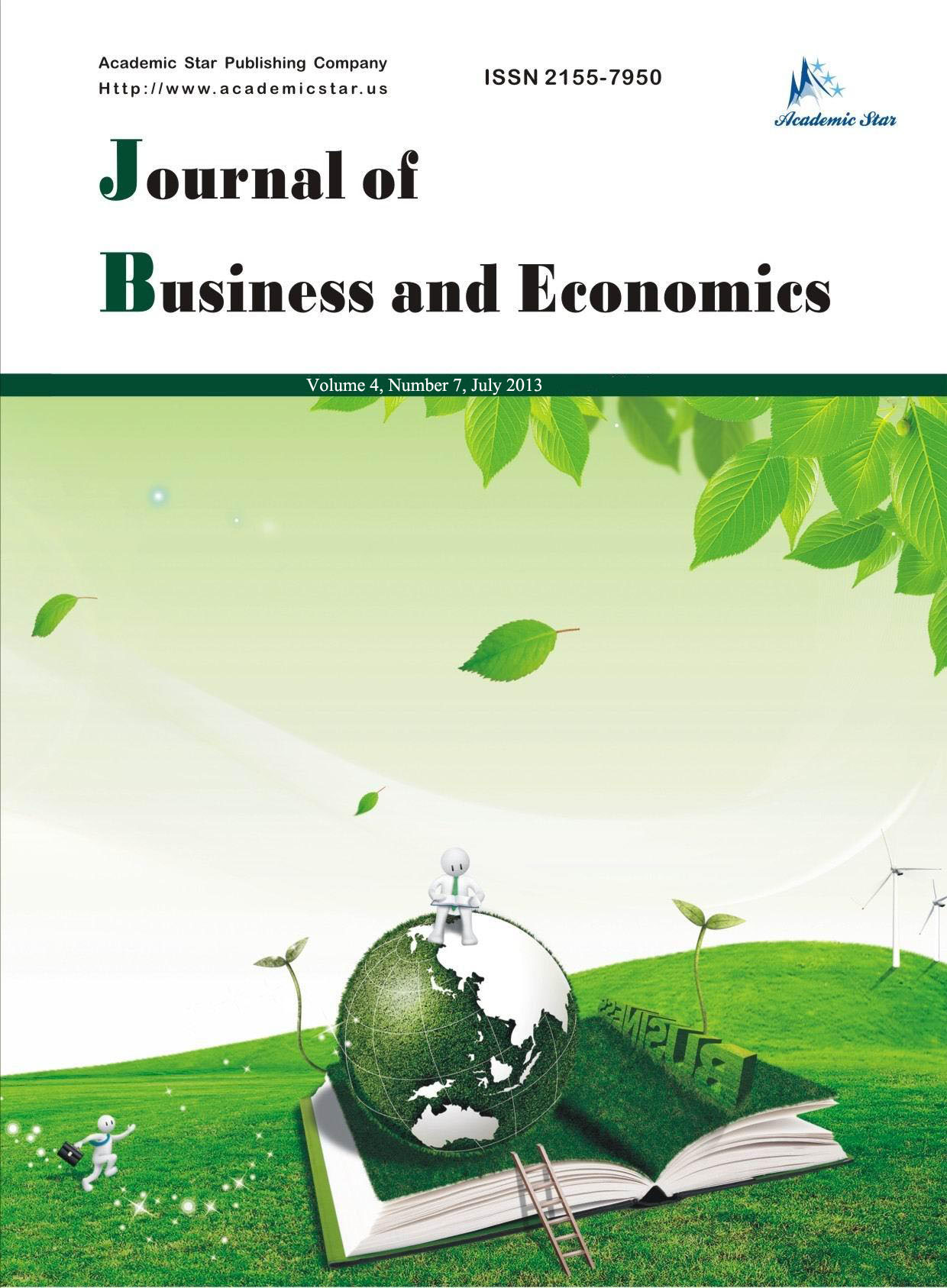
- ISSN: 2155-7950
- Journal of Business and Economics
The Use of Machine Learning to Create Predictive Models for Escrowing Shareholders in the Brazilian Investment Funds
Abstract: This paper seeks to identify the existence of behavioral variables of investment fund shareholders that can help explain the termination of a relationship with the financial institution and seek models with the best performance predictor of dropout. Information from a large Brazilian financial institution is used, totaling 2,907,270 individual customers from the medium to high-income segments and a balanced sample with the under-sampling technique of 200,000 customers. The main variables identified as important in predicting dropout were product ownership, balance and digital profile. After comparing the performance of Receiver Operating Characteristic Curve for the Logistic Regression, Decision Tree, KNN, Naive Bayes, Randon Forest, XGBoost, LDA, MLP and SVC models, the results pointed to XGBoost as the best predictive performance model with Receiver Operating Characteristic curve of 0.7861 and confusion matrix with an accuracy of 71.16.
Key words: investment funds, machine learning, customer evasion, XGBoost, relationship with customers
JEL code: G21, G24, C45, C63